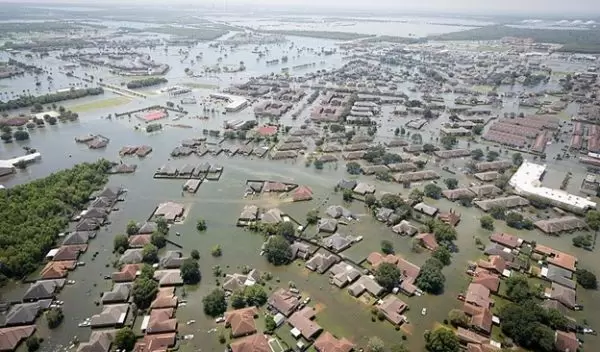
Big data-derived tool facilitates closer monitoring of recovery from natural disasters
By analyzing visitation patterns to essential establishments like pharmacies, religious centers and grocery stores during Hurricane Harvey, researchers at Texas A&M University have developed a framework to assess the recovery of communities after natural disasters in nearly real-time.
The scientists say the information gleaned from their analysis could help agencies allocate resources effectively among communities ailing from a disaster.
"Neighboring communities can be impacted very differently after a natural catastrophic event," said Ali Mostafavi, a civil engineer at Texas A&M University. "We need to identify which areas can recover faster than others, and which areas are impacted more than others so we can allocate resources to areas that need them more."
The U.S. National Science Foundation-funded researchers reported their findings in Interface.
The standard way of obtaining data needed to estimate resilience is through surveys. The questions considered, among others, are how and to what extent businesses or households are affected by the natural disaster and the stage of recovery. However, Mostafavi said these survey-based methods, although extremely useful, take a long time to conduct, with the results becoming available only many months after the disaster.
Mostafavi and collaborators turned to community-level big data, especially information collected from anonymized cell phone data by companies that keep track of visits to locations within a perimeter. In particular, the researchers partnered with a company called SafeGraph to obtain location data for people in Harris County around the time of Hurricane Harvey.
Their analysis revealed that communities that had low resilience also experienced more flooding. However, the results also showed that the level of impact did not necessarily correlate with recovery.
Jacqueline Meszaros, a program director in NSF's Directorate for Engineering, added that, "in addition to being faster than surveys, these research methods avoid some human errors such as memory failure, they have some privacy-preserving advantages, and they don't require time and effort by the people affected. When we can learn about resilience without imposing on those who are still recovering from a disaster, it's a good thing."