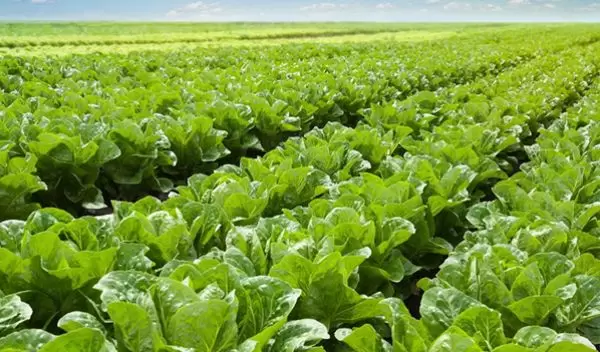
Machine learning can reduce concerns about nanoparticles in food
While crop yield has achieved a substantial boost from nanotechnology in recent years, alarms over the health risks posed by nanoparticles in fresh produce and grains have also increased. Nanoparticles entering the soil through irrigation, fertilizers and other sources have raised concerns about whether plants absorb these minute particles enough to cause toxicity.
In a study published in the journal Environmental Science and Technology, researchers at Texas A&M University used machine learning to evaluate the properties of metallic nanoparticles that make them more susceptible to plant uptake. The researchers say their algorithm could indicate how much plants accumulate nanoparticles in their roots and shoots.
Nanoparticles are a burgeoning trend in several fields, including medicine, consumer products and agriculture. Depending on the type of nanoparticle, some have favorable surface properties, charge and magnetism, among other features. These qualities make them ideal for many applications. For example, in agriculture, nanoparticles may be used as antimicrobials to protect plants from pathogens. Alternatively, they can bind to fertilizers or insecticides and then be programmed for slow release to increase plant absorption.
These agricultural practices and others, like irrigation, can cause nanoparticles to accumulate in the soil. However, with the different types of nanoparticles that could exist in the ground and a staggeringly large number of terrestrial plant species, including food crops, it is uncertain whether certain properties of nanoparticles make them more likely to be absorbed by some plant species than others.
For their study, the U.S. National Science Foundation-funded researchers chose two different machine learning algorithms: an artificial neural network and gene-expression programming. They first trained these algorithms on a database created from past research on different metallic nanoparticles and the specific plants in which they had accumulated. The database contained the size, shape and other characteristics of the nanoparticles, along with information on how many of these particles were absorbed from soil or nutrient-enriched water into the plant body.
"These scientists applied state-of-the art machine learning techniques to a question that is of great interest to society," says Anne-Marie Schmoltner, a program director in NSF's Division of Chemistry.