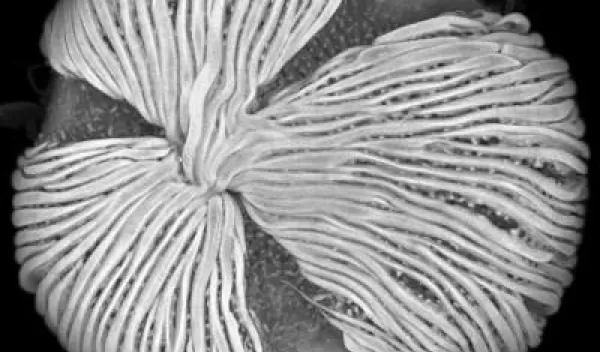
Modern computational tools open new era of fossil pollen research
One of the best sources of information on the evolution of terrestrial ecosystems and plant diversity over millions of years is fossil pollen. For palynologists -- the scientists who study ancient pollen -- a common challenge in the field is the identification of plant species based on fossil grains.
By integrating machine-learning technology with high-resolution imaging, a U.S. National Science Foundation-funded team at the Smithsonian Tropical Research Institute, the University of Illinois at Urbana-Champaign, the University of California, Irvine and collaborating institutions was able to make advances to meeting this challenge. The results were published in Proceedings of the National Academy of Sciences.
To help improve the efficiency and accuracy of fossil pollen identification, scientists developed and trained three machine-learning models to differentiate among several existing Amherstieae legume genera and tested them against fossil specimens from western Africa and northern South America dating back to the Paleocene (56-66 million years ago), Eocene (34-56 million years ago) and Miocene (5.3-23 million years ago).
The models classified existing pollen accurately more than 80% of the time and showed high consensus on the identification of fossil pollen specimens. These results support previous hypotheses suggesting that the Amherstieae originated in Africa and later dispersed to South America, revealing an evolutionary history of nearly 65 million years.
"We do not know the biological affinity of the majority of types of deep-time fossil pollen," said Smithsonian paleontologist Carlos Jaramillo, co-author of the study. "This study shows that with the right tools, we are able to taxonomically classify fossil pollen beyond what has been previously possible."
However, more than a third of the fossil specimens did not present biological affinity with any existing genera, suggesting that part of this ancient diversity may have gone extinct at some point during the evolutionary process.
"These new tools reveal the vast amount of taxonomic information pollen can offer and that has been hidden from researchers until now," said Ingrid Romero at the University of Illinois-Urbana Champaign and lead author of the study.
This new approach improves the taxonomic resolution of fossil pollen identification and greatly enhances the use of pollen data in ecological and evolutionary research. It also narrows down the range of options for experts in fossil pollen identification, allowing them to save time and invest their energy on the most challenging specimens.
"Machine learning and computer vision technologies can not only lead to new scientific discoveries, but also help us better understand what happened in the past," said Jie Yang, a program director in NSF's Directorate for Computer and Information Science and Engineering.