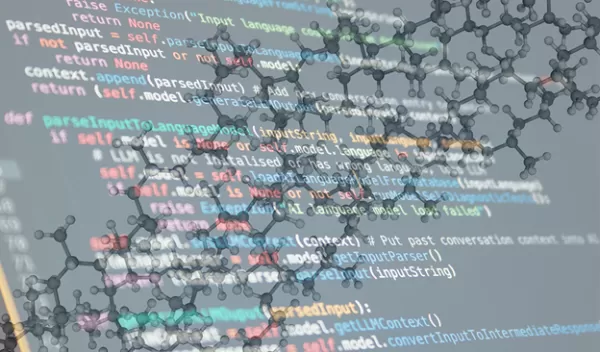
New AI algorithm learns chemical language, accelerates polymer research
Polymers are well-known macromolecules in materials science and engineering communities, but most of us may not be aware of how often we're touching, using and interfacing with these materials. Polymers can be engineered to have desired properties such as flexibility, water resistance or electrical conductivity. Nonstick cookware and construction materials, for example, include the polymers polytetrafluoroethylene and polyvinyl chloride.
Figuring out which combinations of materials will make the most effective polymers is a monumental and time-consuming task because the combinations are essentially endless. Now, researchers at Georgia Tech have developed a machine-learning model that could revolutionize how scientists and manufacturers virtually search the chemical space to identify and develop these all-important polymers. The U.S. National Science Foundation-supported team published its findings in Nature Communications.
The work was conceived and guided by engineer Rampi Ramprasad at Georgia Tech. The new tool aims to overcome the challenges of searching the large chemical space of polymers. Trained on a massive dataset of 80 million polymer chemical structures, polyBERT, as it's called, has become an expert in understanding the language of polymers.
"This is a novel application of language models within polymer informatics," said Ramprasad. "While natural language models may be used to extract materials data from the literature, here, we aim such capabilities at understanding the complex grammar and syntax followed by atoms as they come together to make up polymers."
PolyBERT treats chemical structures and connectivity of atoms as a form of chemical language and uses state-of-the-art techniques inspired by natural language processing to extract the most meaningful information from chemical structures. The tool uses Transformer architecture, used in natural language models, to capture the patterns and relationships and learn the grammar and syntax that occur at the atomic and higher levels in the polymer structure.
Speed is one remarkable advantage of polyBERT. Compared to traditional methods, polyBERT is over two orders of magnitude faster. This high-speed capability makes polyBERT an ideal tool for high-throughput polymer informatics pipelines, the researchers said, allowing for the rapid screening of massive polymer spaces.
With advancements in graphics processing unit technology, the computation time for polyBERT fingerprints is expected to improve even further, according to the researchers.
“Researchers funded by the NSF Partnership for Innovation program are developing a new artificial intelligence tool to overcome the challenge of determining which combinations of chemicals will make the most effective polymers,” says Debora Rodrigues, a program director in NSF’s Directorate for Technology, Innovation and Partnerships. “They’re using AI to train on a massive dataset of 80 million polymer chemical structures, allowing for the rapid screening of diverse polymers without the need of laboratory experimentations.”