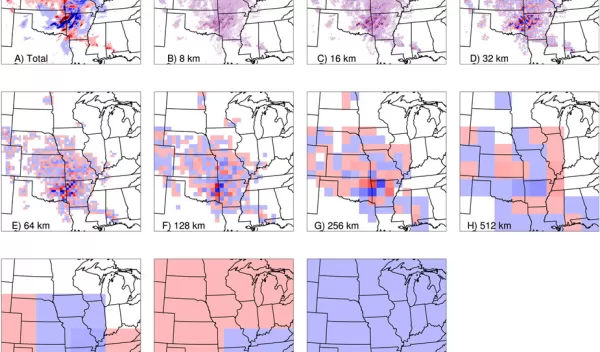
Fine-tuning forecasts of nighttime storms on the plains
The Midwestern plains of the U.S. are known for their dramatic storms: tornadoes, hail, thunderstorms. But one storm feature has mystified researchers: Why does their frequency seem to peak at night?
Xuguang Wang, an associate professor of meteorology at the University of Oklahoma, is part of a team working on the multi-agency PECAN (Plains Elevated Convection At Night) project, designed to advance the understanding -- and prediction -- of nocturnal, warm-season precipitation through improved use of data and simulation.
"Scientists still don't understand mechanics of nighttime storms exactly," Wang says. "There are hypotheses, but there's no consensus about the physical process yet." Moreover, "our skill in predicting where they will form and how strong they'll be at night is poor."
To better comprehend the dynamics of these storms, research teams supported by the National Science Foundation (NSF), the National Oceanic and Atmospheric Administration (NOAA), NASA and the Department of Energy have been collecting data before and during nighttime thunderstorms in the arid western Great Plains to learn what triggers these storms, how the atmosphere supports their lifecycle, and how they impact lives, property, agriculture and the water budget in the region.
"During the field campaign, there are a lot of people collecting observations to help us understand nocturnal storms and improve the forecasts," Wang said.
But how do the teams know where to set up their operations to best observe the storm? They rely on numerical weather forecasts that use computer models and simulations to provide predictions on atmospheric conditions such as temperature, pressure, wind and rainfall.
Over 45 days in June and July 2015, Wang and her team used the Stampede supercomputer at The University of Texas at Austin's Texas Advanced Computing Center to run real-time forecasts of the night storms to help people who were going to deploy the measuring instruments know where to place them.
More than 35 different science vehicles and instruments were used to measure different aspects of the atmospheric conditions. They included the Doppler on Wheels (DOW), Mobile Meso-nets and several aircrafts from NASA and NOAA. Based on the daily numerical forecasts produced by Wang's and other teams, they decided where to place their observing vehicles at night for maximum data gathering.
Data-driven advances in weather forecasting
Numerical weather prediction has been around since the 1970s and has increased in accuracy as weather models have improved and computers have grown faster and more powerful.
However, forecasts by Wang's team go beyond traditional numerical predictions by using an approach called data assimilation, where real-time measurements from the field are fed into the prediction models as they compute -- rather than only at the outset -- to provide a more accurate assessment of where a storm might form.
In the PECAN project, this creates a feedback loop whereby data assimilation improves numerical weather predictions by Wang's team, which in turn improves the observing teams' ability to capture useful, precise observations.
Creating these data-rich forecasts was extremely computer-intensive. To get an accurate estimate of where storms might emerge, her team had to run an ensemble of forecasts -- a weather prediction method that uses many forecasts, with different starting conditions and different ways of representing complex processes, all simulated in parallel, to generate a representative sample of the possible future states of a dynamic system. (Think of the multi-line hurricane tracks commonly shown as a storm approaches landfall.)
Wang and her team produced two forecasts a day up to 48 hours in advance of a storm. For the 24 hour forecast, the system generated 20 possible outcomes, and for day two, it predicted 10 outcomes.
To calculate the atmospheric conditions evolving over time on a 3-D grid, each simulation utilized more than 1,000 computer processors in parallel on Stampede, the equivalent of several hundred individual computers working in tandem. This assured the forecasts were available in time to make decisions about the deployment of the measuring instruments later in the day.
Computing time on Stampede was allocated through the Extreme Science and Engineering Discovery Environment (XSEDE) and accounted for millions of computing hours in total.
"There were some cases where we know we did a good job and there were some cases where we know we didn't," Wang said. "There is an intrinsic low-predictability of some of the cases, so it will be interesting to explore when we were successful and when we weren't and why."
Post-analysis helps determine best ways to use data
Even after the field campaign, Wang and her team continue to use Stampede to further analyze forecasts with a goal of identifying the factors that cause a storm to occur and grow and incorporating that understanding into future predictions.
"Nocturnal convection has multiscale features, which is to say the trigger of the system can be dependent on features at multiple scales," Wang says.
Because of the intensive observations, various scales of the phenomenon were well sampled -- from the regional environment to the fine-grained features of the storm itself. But how does one determine which aspect of the data is most critical to predicting whether a storm will form?
Analyzing the ensemble forecasts after the fact, Wang tried to determine how various features influenced the accuracy of the forecasts in past cases and then had the computer assign weights to these features for future forecasts.
"The simulations give us the cases to use to see how the system performed and to take a deeper look at how to improve our prediction and understanding and how to improve the model configuration in terms of the resolution and number of vertical levels," she says. "From this, we determine the optimum way to assimilate data in the future."
Chungu Lu, an NSF program officer in the Division of Atmospheric and Geospace Sciences, says this data-driven approach is critical for understanding a wide range of forecasting problems.
"Understanding storm development and formation needs two indispensable research efforts: observing and collecting data in the real world during a storm event; and putting the observational data into some diagnostic or prognostic models," Lu said.
The latter part, nowadays, is often carried out on computers that crunch this data and simulate different solutions that scientists use to test their hypotheses and predictions.
"In this sense, machines aid our brains to understand a physical phenomenon."
Wang's team presented their research results at the 37th Conference on Radar Meteorology in September 2015.
"It took quite a bit of human effort," Wang said. "We were working very hard during the field campaign to make sure that the forecasts were available. But as a result, it's going to improve our understanding and also provide guidance in the future."