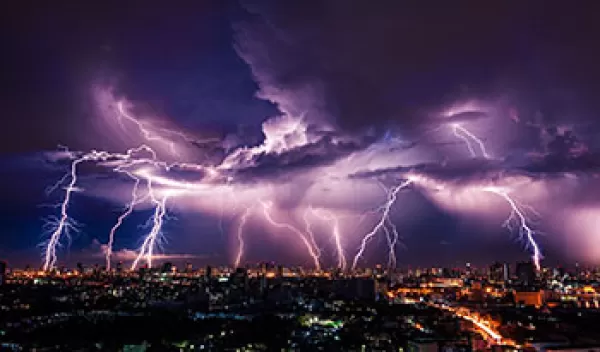
Using artificial intelligence to better predict severe weather
When forecasting weather, meteorologists use a number of models and data sources to track shapes and movements of clouds that could indicate severe storms. However, with increasingly expanding weather data sets and looming deadlines, it is nearly impossible for them to monitor all storm formations -- especially smaller-scale ones -- in real time.
Now, there is a computer model that can help recognize severe storms more quickly and accurately, thanks to a team of researchers partially funded by the National Science Foundation.
The researchers from Penn State, AccuWeather, Inc. and the University of Almería in Spain developed a framework based on machine learning linear classifiers -- a kind of artificial intelligence -- that detects from satellite images rotational movements in clouds that might have otherwise gone unnoticed.
"The very best forecasting incorporates as much data as possible," said Steve Wistar, senior forensic meteorologist at AccuWeather. "There’s so much to take in, as the atmosphere is infinitely complex."
The results of the project, reported in a Penn State press release and published in IEEE Transactions on Geoscience and Remote Sensing, enhance earlier work between AccuWeather and a Penn State College of Information Sciences and Technology research group led by professor James Wang.
"We recognized when our collaboration began [with AccuWeather in 2010] that a significant challenge facing meteorologists and climatologists was in making sense of the vast and continually increasing amount of data generated by Earth observation satellites, radars and sensor networks," said Wang. "It is essential to have computerized systems analyze and learn from the data."
NSF funds Wang’s research through a Computer and Information Science and Engineering CAREER award, whose goal is to develop an interdisciplinary research and education program for investigating the theoretical and computational principles of machine-learning-based image annotation and retrieval.